This is the official implementation for: Topological-Preserving Membrane Skeleton Segmentation in Multiplex Immunofluorescence Imaging.
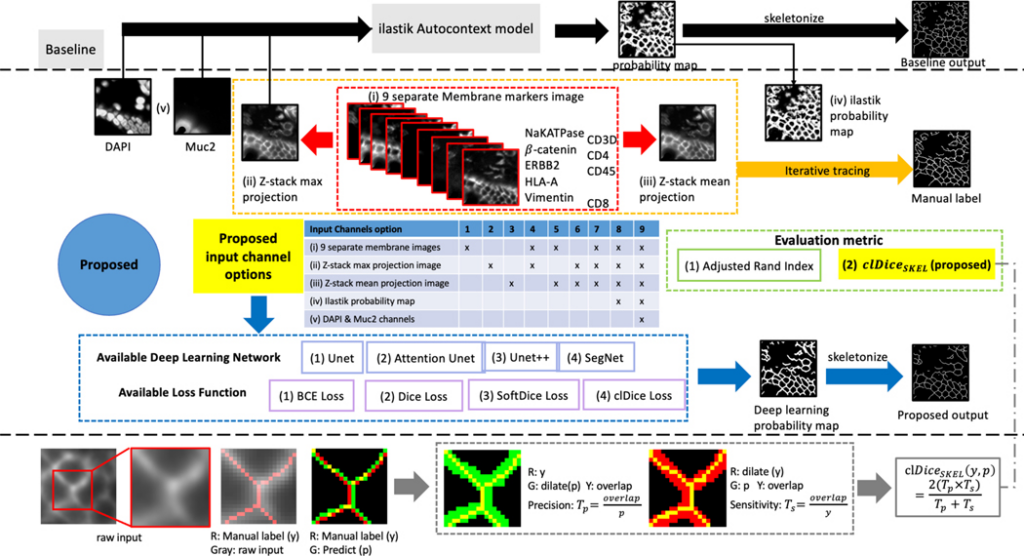
Source code repository
https://github.com/MASILab/MxIF_Membrane_Segmentation
Get our docker image
To do
Run Code
To do
Description
Multiplex immunofluorescence (MxIF) is an emerging imaging technology whose downstream molecular analytics highly rely upon the effectiveness of cell segmentation. In practice, multiple membrane markers (e.g., NaKATPase, PanCK and β-catenin) are employed to stain membranes for different cell types, so as to achieve a more comprehensive cell segmentation since no single marker fits all cell types. However, prevalent watershed-based image processing might yield inferior capability for modeling complicated relationships between markers.
The main contributions of this work are: (1) We develop and comprehensively evaluate the deep membrane segmentation frameworks for large-scale MxIF multi-channel data. (2) We propose a novel metric that is topology preserving and skeleton-based, denoted as 𝑐𝑙𝐷𝑖𝑐𝑒𝑆𝐾𝐸𝐿 to fill the gap of lacking objective metrics for membrane skeleton segmentation. (3) We perform the first deep learning membrane segmentation study with large-scale MxIF markers.
Reference
Topological-Preserving Membrane Skeleton Segmentation in Multiplex Immunofluorescence Imaging
Shunxing Bao, Can Cui, Jia Li, Yucheng Tang, Ho Hin Lee, Ruining Deng, Lucas W Remedios, Xin Yu, Qi Yang, Sophie Chiron, Nathan Heath Patterson, Ken S Lau, Qi Liu, Joseph T Roland, Lori A Coburn, Keith T Wilson, Bennett A Landman, Yuankai Huo
SPIE Medical Imaging 2023