This is the official implementation for: Nucleus subtype classification using inter-modality learning. The data related to Hematoxylin and eosin (H&E) whole slide imaging and multiplexed immunofluorescence (MxIF) imaging.
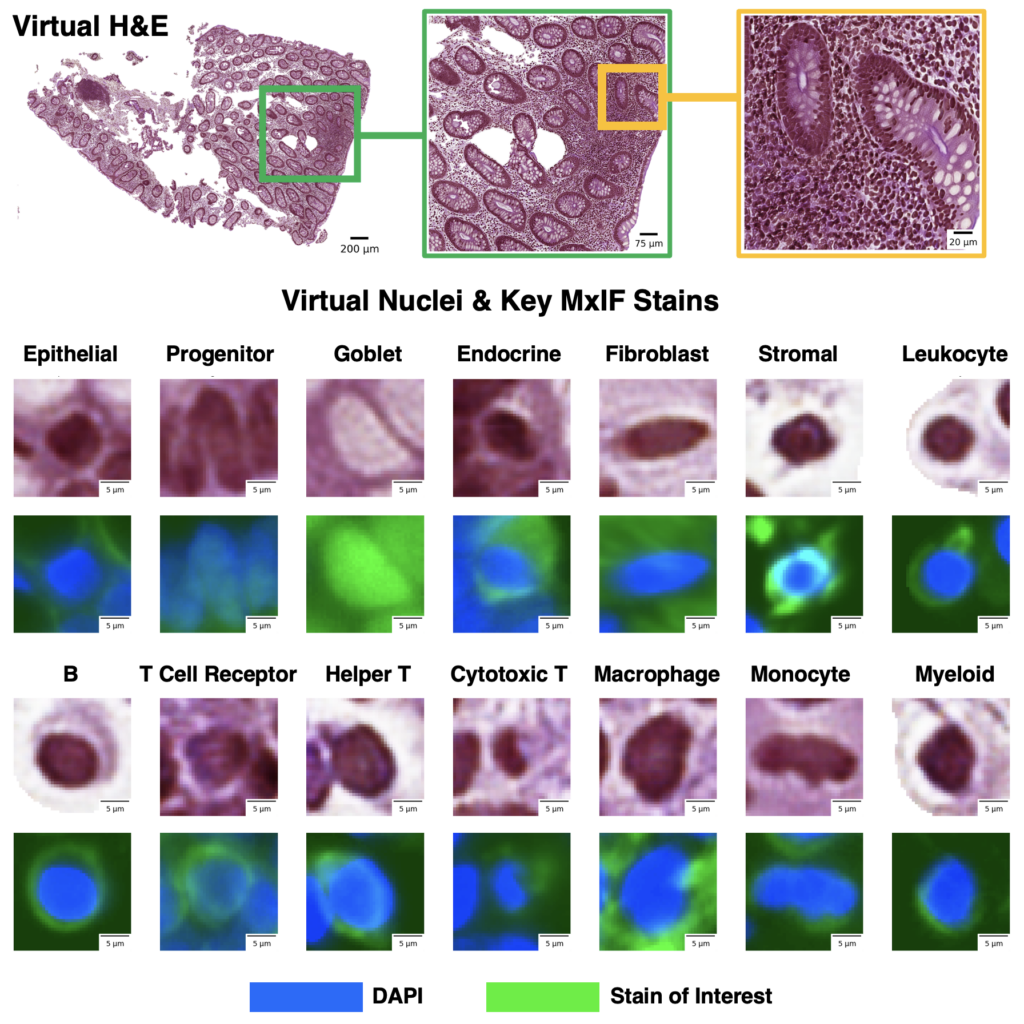
Source Code Repository
Get our docker image
Todo
Run code
Todo
Description
Understanding the way cells communicate, co-locate, and interrelate is essential to understanding human physiology. Hematoxylin and eosin (H&E) staining is ubiquitously available both for clinical studies and research. The Colon Nucleus Identification and Classification (CoNIC) Challenge has recently innovated on robust artificial intelligence labeling of six cell types on H&E stains of the colon. However, this is a very small fraction of the number of real cell types. Specifically, the CoNIC Challenge is unable to classify epithelial subtypes (progenitor, endocrine, goblet), lymphocyte subtypes (B, helper T, cytotoxic T), or connective subtypes (fibroblasts, stromal). In this paper, we propose to use inter-modality learning to label previously un-labelable cell types on virtual H&E. We leveraged multiplexed immunofluorescence (MxIF) histology imaging to identify 14 subclasses of cell types. We performed style transfer to synthesize virtual H&E from MxIF and transferred the higher density labels from MxIF to these virtual H&E images. We then evaluated the efficacy of learning in this approach. We identified helper T and progenitor nuclei with positive predictive values of 0.34 ± 0.15 (prevalence 0.03 ± 0.01) and 0.47 ± 0.1 (prevalence 0.07 ± 0.02) respectively on virtual H&E. This is a promising step towards automating annotation in digital pathology.
The main contribution of this work is to enable the first approach to automatically identify helper T and progenitor nuclei on virtual H&E.
Reference
Nucleus subtype classification using inter-modality learning.
Lucas W. Remedios, Shunxing Bao , Samuel W. Remedios, Ho Hin Lee , Leon Y. Cai , Thomas Li, Ruining Deng, Can Cui, Jia Li, Qi Liu, Ken S. Lau, Joseph T. Roland, Mary K. Washington, Lori A. Coburn, Keith T. Wilson, Yuankai Huo, Bennett A. Landman
Accepted SPIE Medical Imaging 2024